Contents
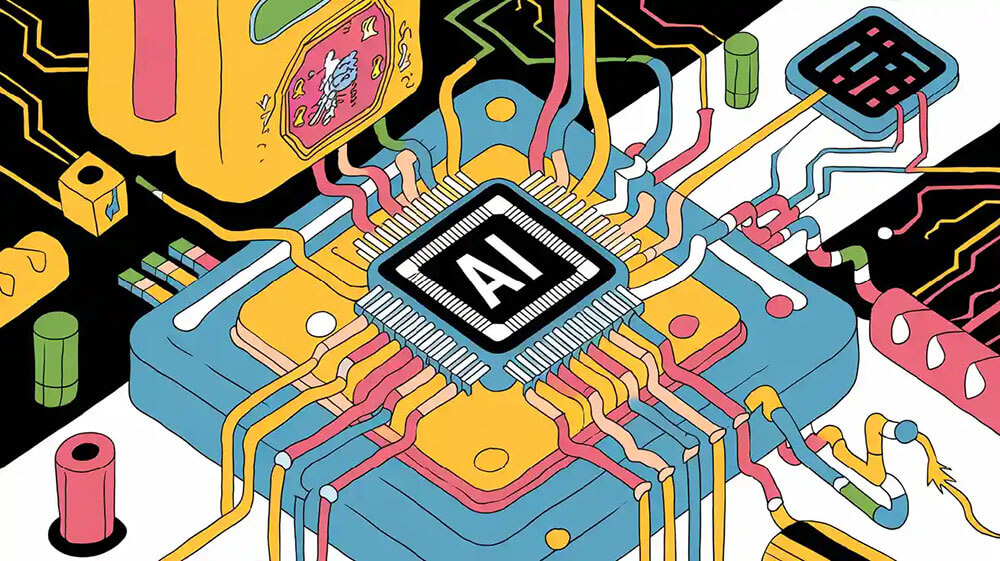
AI is revolutionizing the way you manage lithium-ion batteries, making them safer, more efficient, and sustainable. By leveraging AI-driven BMS of lithium battery technology, industries like medical, robotics, and infrastructure are witnessing groundbreaking advancements. For example:
AI-driven anomaly detection reduces fire risks by 70%, enhancing safety.
Adaptive charging algorithms cut battery degradation by 30%, extending lifespan.
Optimized recycling techniques recover 95% of lithium, boosting sustainability.
These innovations are reshaping energy storage and management, paving the way for a smarter future.
Key Takeaways
AI-powered battery systems make lithium batteries 70% safer. This lowers fire risks and improves safety for many uses.
Predictive tools check battery health, so you can replace them on time and prevent sudden failures.
Real-time adjustments help batteries work better and last longer by adapting to changes.
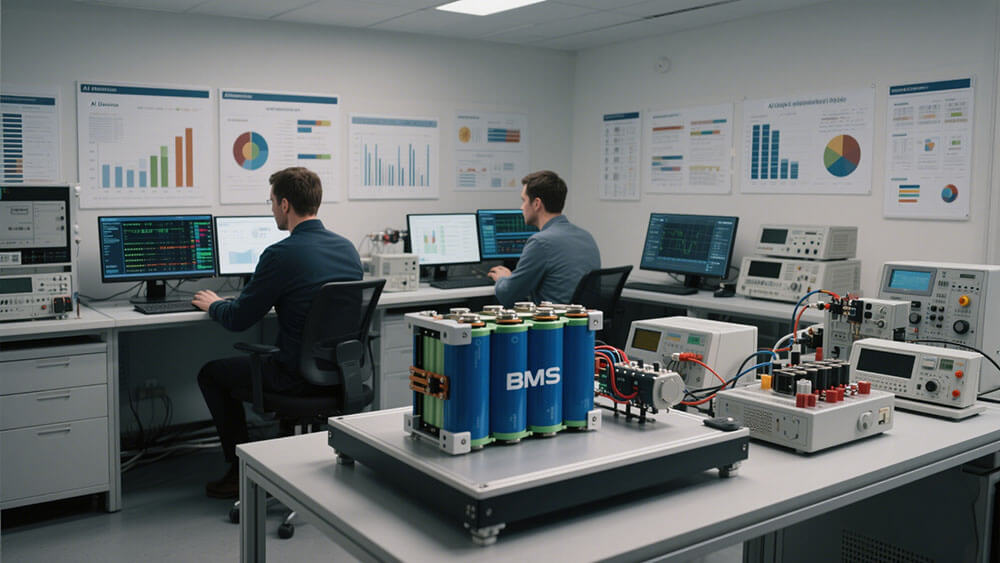
Part 1: Defining AI-Driven BMS of Lithium Battery
1.1 Overview of Battery Management Systems (BMS)
Battery management systems (BMS) are critical components in modern energy storage solutions. They monitor and regulate the performance of lithium-ion batteries, ensuring safety, efficiency, and longevity. A robust BMS oversees key parameters such as voltage, current, temperature, and state of charge (SOC). These systems also provide protection against overcharging, over-discharging, and overheating, which are common causes of battery failure.
Incorporating advanced technologies, BMS configurations now support both grid-connected and standalone applications. They are compatible with various battery types, including lithium-ion, flow, and sodium-β chemistries. The integration of AI-driven BMS of lithium battery technology has further enhanced their capabilities, enabling real-time data analysis and predictive maintenance.
1.2 Limitations of Traditional BMS Approaches
Traditional BMS approaches face several challenges. Existing models often struggle with inaccuracies due to the nonlinear behavior of lithium-ion batteries. Long-term predictions remain a significant hurdle, conflicting with the industry’s demand for extended battery life. Moreover, traditional systems fail to account for complex dependencies within macro and micro scales, complicating parameterization.
AI-driven BMS of lithium battery technology addresses these limitations by leveraging machine learning algorithms. These algorithms predict energy demand fluctuations, optimize charging cycles, and enable smart grids to adjust energy flow in real time. Research from Stanford demonstrates that AI can predict battery lifespan with 95% accuracy, showcasing its transformative potential.
Challenge | Description |
---|---|
Model Inaccuracy | Existing models have inaccuracies due to the nonlinearity of governing physics and chemistry. |
Long-Term Predictions | Current models struggle with long-term predictions, conflicting with the demand for increased battery life. |
Complex Dependencies | The model must account for dependencies within macro and micro scales, complicating parameterization. |
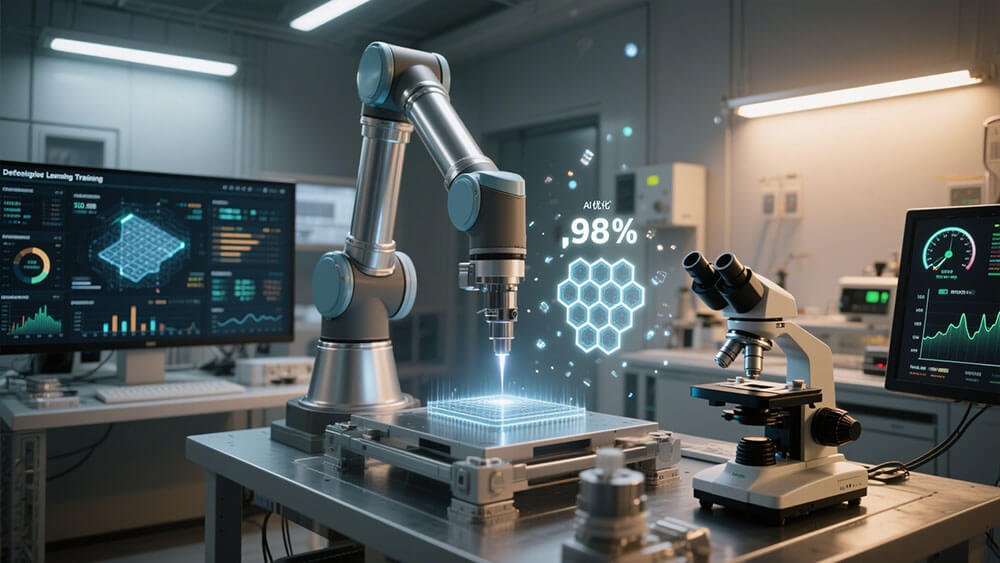
Part 2: AI-Driven Trends in Battery Technology
2.1 Predictive Analytics for Battery Health
Predictive analytics is transforming how you monitor and maintain lithium-ion batteries. By leveraging advanced algorithms, you can track critical parameters such as state of charge (SOC), state of health (SOH), voltage, current, and temperature. This approach enables accurate estimation of a battery’s remaining useful life (RUL), allowing you to schedule timely replacements and avoid unexpected downtimes.
Predictive analytics optimizes charging and discharging cycles, enhancing battery lifespan and efficiency.
Machine learning models analyze usage patterns to recommend optimal charging rates, improving charging efficiency and reducing wear.
For example, in electric vehicles, predictive analytics ensures that batteries operate at peak performance, minimizing degradation during high-demand scenarios. This innovation is particularly valuable in industries like medical devices, where uninterrupted power is critical.
2.2 Real-Time Optimization of Lithium-Ion Batteries
Real-time optimization is a game-changer in battery technology. AI-driven technologies enable dynamic adjustments to battery operations, ensuring maximum efficiency and longevity. For instance, in electric vehicles, real-time optimization balances energy distribution during varying driving conditions, enhancing overall performance. AI-powered design ensures that lithium-ion batteries adapt to changing conditions, making them ideal for applications in robotics and infrastructure.
2.3 AI in Second-Life Battery Management
AI is revolutionizing second-life battery management, offering cost-effective and sustainable solutions. Second-life batteries, repurposed from electric vehicles or other applications, provide a viable alternative for energy storage systems.
Cost savings: Second-life batteries cost $0–60 per kWh, compared to $90–120 per kWh for new batteries.
Environmental benefits: Using one megawatt-hour of second-life batteries reduces embodied carbon by approximately 450 metric tons.
However, challenges remain. Repurposing involves complex processes like screening, tear-down, and testing, which require significant resources. AI-driven technologies streamline these processes, improving efficiency and reducing costs. This approach supports sustainability goals, particularly in industrial and consumer electronics sectors. Learn more about sustainability initiatives here.
2.4 Machine Learning for Fault Detection
Machine learning enhances fault detection in lithium battery systems, ensuring safety and reliability. By analyzing vast datasets, machine learning models identify anomalies and predict potential failures with high accuracy.
A study using a random forest classifier achieved a fault detection accuracy of 97%, identifying features related to internal short circuits.
Other research demonstrates the effectiveness of various machine learning models in diagnosing faults, reducing risks in critical applications like security systems.
This technology is particularly valuable in environments where safety is paramount, such as security systems and medical devices. Explore security system battery solutions here.
2.5 AI-Enhanced Thermal Management Systems
Thermal management is crucial for maintaining the performance and safety of lithium-ion batteries. AI-enhanced systems monitor and regulate temperature in real time, preventing overheating and thermal runaway.
Solid-state batteries, known for their high energy density (300–500 Wh/kg), benefit significantly from AI-driven thermal management. These systems ensure that batteries operate within safe temperature ranges, extending their lifespan and improving reliability.
Applications in infrastructure, such as transportation and energy grids, rely on these advancements to meet growing energy demands. AI-powered thermal management also supports innovation speed in developing next-generation battery technologies. Learn more about infrastructure battery solutions here.
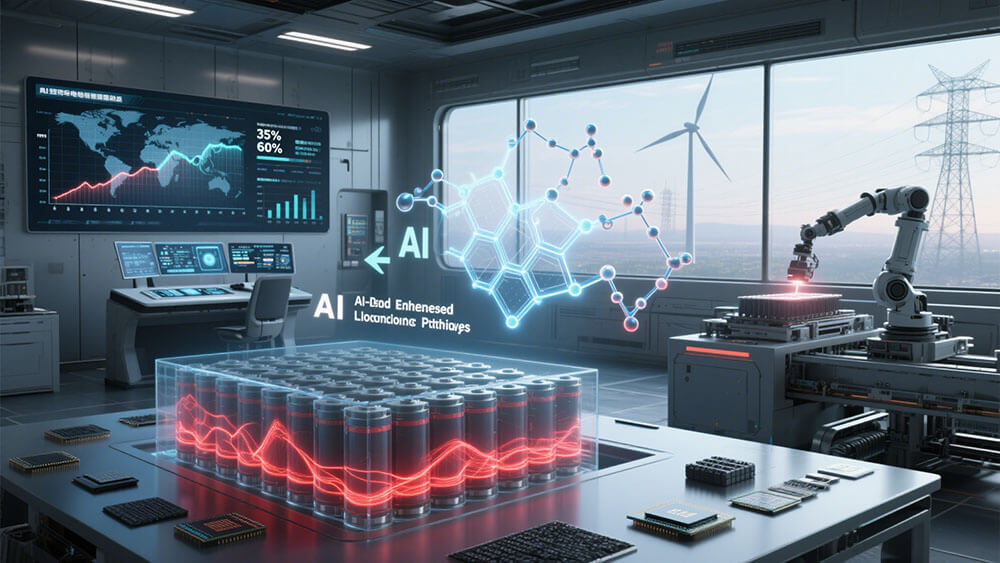
Part 3: The Future of AI in Battery Technology
3.1 Challenges in Scaling AI-Driven BMS
Scaling AI-driven battery management systems (BMS) presents several challenges. The battery industry faces hurdles in integrating AI technologies into existing systems due to high costs and complex infrastructure requirements. For instance, implementing AI in lithium-ion batteries demands advanced hardware and software, which increases production expenses. Additionally, ensuring compatibility across diverse applications, such as robotics and infrastructure, complicates the process.
Sustainability is another critical concern. While AI optimizes battery performance and extends lifespan, the energy density of current lithium-ion batteries still limits their efficiency in large-scale applications. Addressing these limitations requires significant research and development investments. Reports indicate that by 2035, AI will play a pivotal role in cell development and manufacturing, particularly for renewable energy and electric vehicles. However, achieving this vision necessitates overcoming scalability and cost barriers.
Report Title | Key Insights | Expected Growth |
---|---|---|
AI-Driven Battery Technology Market Size, Growth Report by 2034 | Significant growth driven by demand in EVs, renewable energy, and consumer electronics. | Notable CAGR from 2025 to 2034 |
AI Is Well Set to Disrupt the Battery Supply Chain and Life Cycle | AI will be common in cell development and manufacturing, especially for energy storage and EVs. | AI applications will address electrification needs by 2035 |
The Future of Battery Technology: 2030 Market Predictions and Energy Storage Innovations | 90% of battery systems will use AI for optimization by 2030, enhancing battery health and efficiency. | Integration of AI in BMS by 2030 |
3.2 Vision for Fully Autonomous Battery Systems
The future of the battery market lies in fully autonomous systems. AI technologies, such as predictive analytics and reinforcement learning, are key to achieving this vision. Predictive maintenance, powered by machine learning, can forecast failures and recommend preventive measures, ensuring uninterrupted operations. For example, real-time anomaly detection in lithium-ion batteries monitors voltage, current, and temperature to prevent risks like thermal runaway.
Dynamic optimization of charging and discharging patterns based on user behavior further enhances battery performance. By 2030, 90% of battery systems are expected to utilize AI-driven optimization, significantly improving energy density and efficiency. This advancement will support the growing demand for renewable energy and sustainable energy solutions.
Fully autonomous battery systems will also address challenges in scalability and sustainability. Emerging technologies, such as solid-state batteries, promise higher energy density and longer lifespans, making them ideal for industrial and consumer electronics applications. AI will enable these systems to adapt to unexpected conditions, ensuring reliability across diverse sectors, including transportation and medical devices.
The integration of AI in battery energy storage systems represents a transformative step toward sustainable energy solutions. As the battery industry evolves, embracing AI-driven innovations will unlock new potentials in energy storage and management.
AI has revolutionized how you manage lithium-ion batteries. It enhances safety, optimizes charging, and delivers sustainable solutions. By predicting battery health and enabling real-time adjustments, AI ensures efficiency and reliability. Future advancements will unlock fully autonomous systems, transforming energy storage and driving innovation across industries.
FAQ
1. What is the role of AI in improving battery performance?
AI enhances battery performance by optimizing charging cycles, predicting energy demand, and managing thermal conditions. These improvements ensure longer lifespan and better energy storage efficiency.
2. How does AI contribute to second-life battery applications?
AI streamlines second-life battery processes by automating screening, testing, and repurposing. This reduces costs and supports sustainable energy storage solutions for industrial and consumer applications.
Tip: For professional guidance on second-life battery, visit Large Power.