Contents
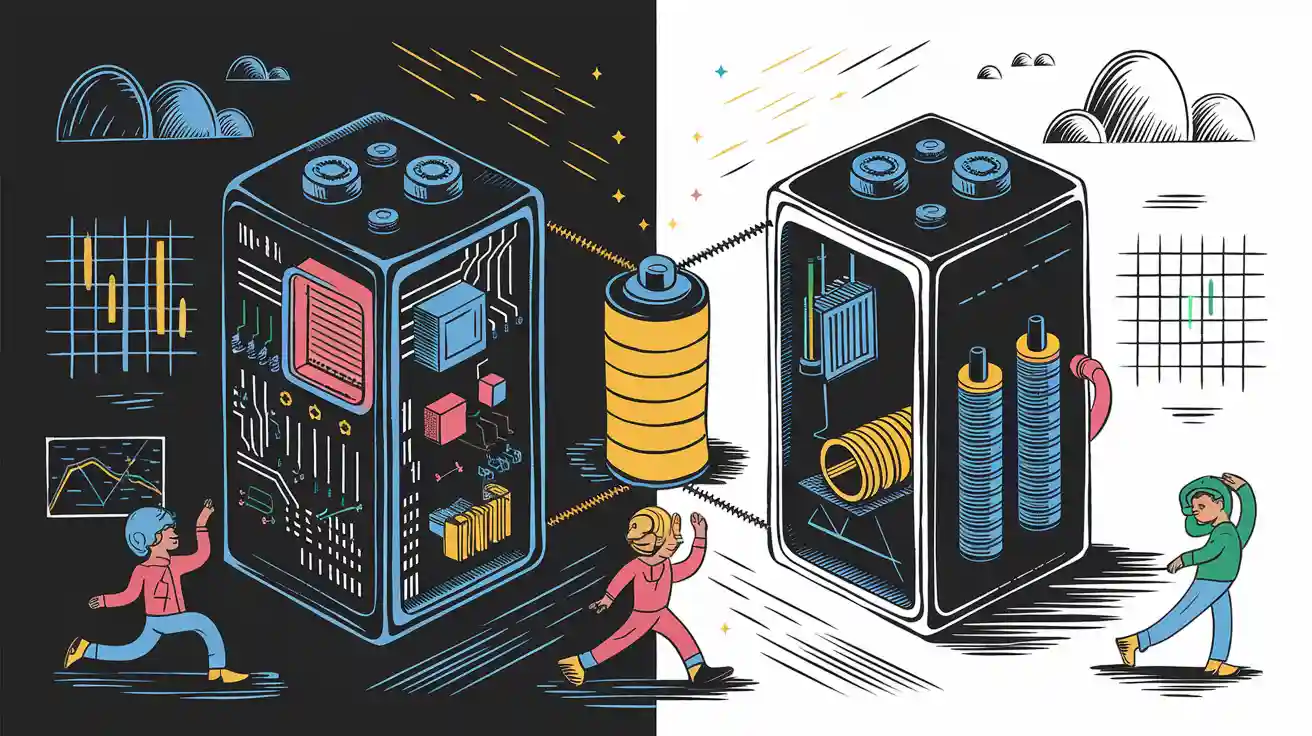
The SOH of lithium battery refers to the state of health, which indicates the remaining capacity or performance in relation to its original condition. Accurate monitoring of the SOH of lithium battery is crucial for predicting battery lifespan and ensuring safety, particularly in industrial applications. Methods for estimating the SOH of lithium battery can be categorized into two types: data-driven approaches that depend on historical data patterns, and model-based techniques that utilize mathematical representations of battery behavior. Understanding these methods allows you to choose the most appropriate option for optimizing performance and cost-efficiency.
Key Takeaways
Data-driven methods are great for quick updates and flexibility. They work well in things like electric cars and robots.
Model-based methods are very accurate and show battery wear. They are best for safe places like medical tools.
Hybrid methods mix both types, giving a good balance. They are useful for tricky tasks needing both accuracy and flexibility.
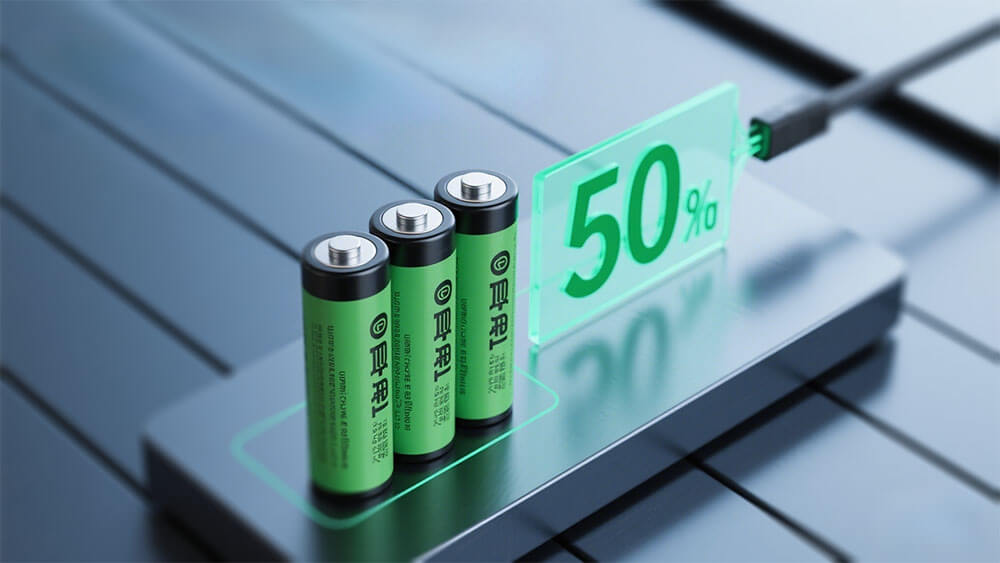
Part 1: Overview of SOH Estimation Methods
1.1 Definition of SOH for Lithium-Ion Batteries
The state of health (SOH) for lithium-ion batteries measures the current capacity or performance compared to its original state. This metric, expressed as a percentage, helps you understand how much of the battery’s initial functionality remains. Regulatory standards, such as the California Advanced Clean Cars II initiative, emphasize the importance of standardized SOH indicators. These indicators aim to improve transparency and trust in battery health information for electric vehicles.
1.2 Importance of SOH Estimation in Battery Management Systems
SOH estimation plays a critical role in battery management systems (BMS). Accurate monitoring of the state of health ensures optimal performance, safety, and longevity of lithium-ion batteries. A reliable BMS uses SOH data to prevent overcharging, overheating, and other risks that could compromise battery integrity. For industries relying on large-scale battery systems, such as robotics or infrastructure, precise SOH estimation minimizes downtime and maintenance costs.
1.3 Applications of SOH Estimation in Industry
SOH estimation finds applications across various industrial sectors. For example, in robotics, it ensures uninterrupted operation by predicting battery replacement needs. In infrastructure, such as transportation systems, it supports efficient energy management. Empirical studies, like those using NASA’s lithium-ion battery datasets, highlight the importance of SOH estimation in real-world scenarios. These studies focus on discharge processes to predict battery health and performance.
Parameter | Description |
---|---|
Dataset | NASA lithium-ion battery charge and discharge experimental data set |
Battery Numbers | B7, B6, B5 (all rated at 2 Ah) |
Charging Conditions | Charged to 4.2 V at 1.5 A, then in constant voltage mode until current dropped to 20 mA |
Discharge Conditions | Discharged at 2 A until voltage dropped to specified levels (2.2 V, 2.7 V, 2.5 V) |
Temperature | Experiments conducted at room temperature (24˚C) |
Performance Measurement | Stopped when actual capacity fell below 70% of Acceptable Performance Ratio capacity |
Focus | Emphasizes the discharge process for predicting and estimating SOH of batteries |
For customized solutions tailored to your industrial needs, explore Large Power’s custom battery solutions.
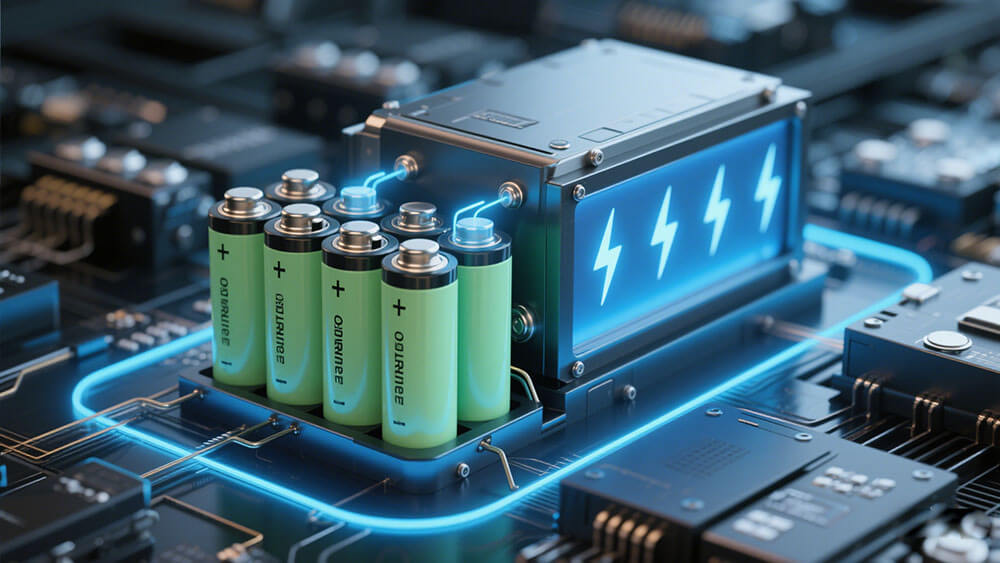
Part 2: Data-Driven SOH Estimation Methods
2.1 Principles of Data-Driven Methods
Data-driven methods rely on historical and real-time data to estimate the state of health (SOH) of lithium-ion batteries. These methods utilize machine learning algorithms and statistical models to identify patterns in battery degradation. By analyzing parameters such as voltage, current, and temperature, data-driven prediction models can assess battery capacity and performance without requiring detailed knowledge of internal electrochemical processes.
For example, advanced techniques like support vector regression (SVR) and neural networks, including CNN-LSTM combinations, have demonstrated significant accuracy in SOH estimation. These algorithms excel at processing time-series data, making them ideal for tracking battery degradation over time. The adaptability of these methods allows them to handle diverse datasets, ensuring robust performance across various applications.
2.2 Advantages of Data-Driven SOH Estimation
Data-driven SOH estimation offers several benefits:
No dependency on electrochemical modeling: These methods eliminate the need for complex internal battery models, simplifying implementation.
Enhanced accuracy and efficiency: Techniques like SVR and CNN-LSTM combinations optimize SOH prediction, reducing errors such as RMSE and MAE.
Adaptability to real-world datasets: Data-driven approaches perform effectively even with noisy or incomplete data, as demonstrated in studies by Lin et al. and Ren et al.
Method | Advantages | Performance Metrics |
---|---|---|
Data-driven methods | No need for internal modeling | Improved accuracy, efficiency, adaptability |
Support Vector Regression | Optimized SOH estimation parameters | Enhanced prediction of remaining useful life |
CNN and LSTM combination | Effective on real-world datasets | Significant accuracy improvements, reduced RMSE and MAE |
2.3 Limitations of Data-Driven Methods
Despite their advantages, data-driven methods face challenges:
Dependency on data quality: The accuracy of SOH estimation depends heavily on the quality and quantity of the data used.
Complex feature extraction: Identifying features that correlate strongly with battery degradation often requires extensive preprocessing.
Challenges in model construction: Building models for time-series data can be difficult due to the varying importance of information across sequences.
To overcome these limitations, you can explore hybrid approaches that combine data-driven and model-based methods for improved SOH estimation. For tailored solutions, consider consulting Large Power’s custom battery solutions.
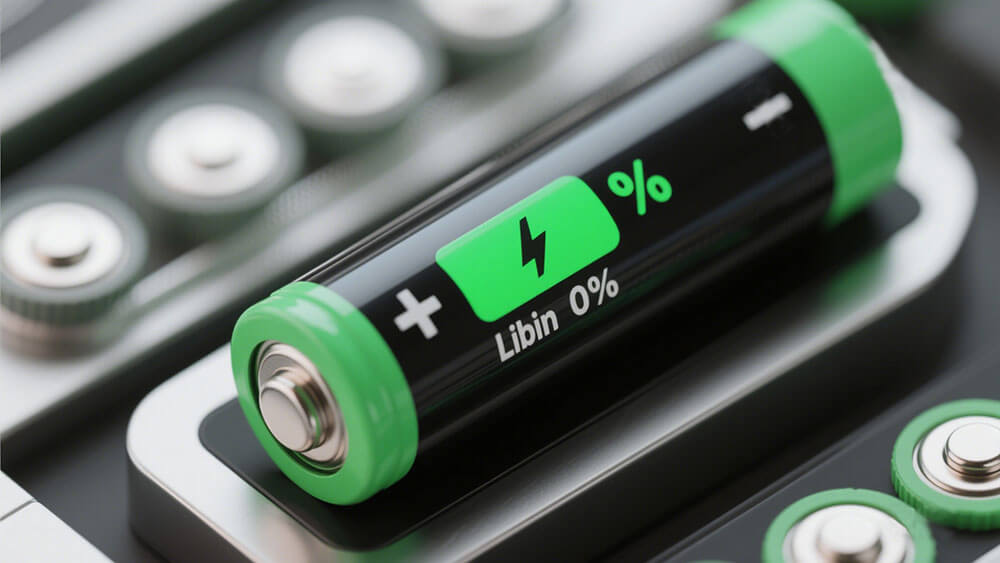
Part 3: Model-Based SOH Estimation Methods
3.1 Principles of Model-Based Methods
Model-based methods for state of health estimation rely on mathematical and physical models to simulate the internal behavior of lithium-ion batteries. These models incorporate parameters such as voltage, current, temperature, and capacity to predict battery degradation. By understanding the electrochemical processes, you can achieve precise SOH estimation.
One notable approach involves physics-informed neural networks, which combine physical modeling with machine learning. For instance, a study titled Physics-informed neural network for lithium-ion battery degradation stable modeling and prognosis demonstrated the effectiveness of this method. Using a dataset of 387 batteries with over 310,000 samples, the model achieved a mean absolute percentage error (MAPE) of just 0.87% in SOH estimation. This highlights the potential of integrating physics-based insights with advanced algorithms to enhance accuracy.
3.2 Advantages of Model-Based SOH Estimation
Model-based methods offer several benefits for SOH estimation:
High accuracy: These methods provide precise predictions by simulating the internal dynamics of lithium-ion batteries.
Robustness: They perform well under varying operating conditions, making them suitable for industrial applications.
Insight into degradation mechanisms: By modeling physical processes, you gain a deeper understanding of battery degradation, which aids in optimizing battery design and usage.
These advantages make model-based methods a preferred choice for applications requiring detailed analysis, such as medical devices or robotics.
3.3 Limitations of Model-Based Methods
Despite their strengths, model-based methods face challenges:
The complexity of physical models makes them difficult to implement.
Multiple parameters, such as charge-discharge currents and temperature, significantly affect battery performance.
Developing accurate electrochemical models involves high costs, limiting their widespread application.
Tip: To overcome these limitations, consider hybrid approaches that combine model-based and data-driven methods. These approaches leverage the strengths of both techniques, ensuring better estimation performance. For tailored solutions, explore Large Power’s custom battery solutions.
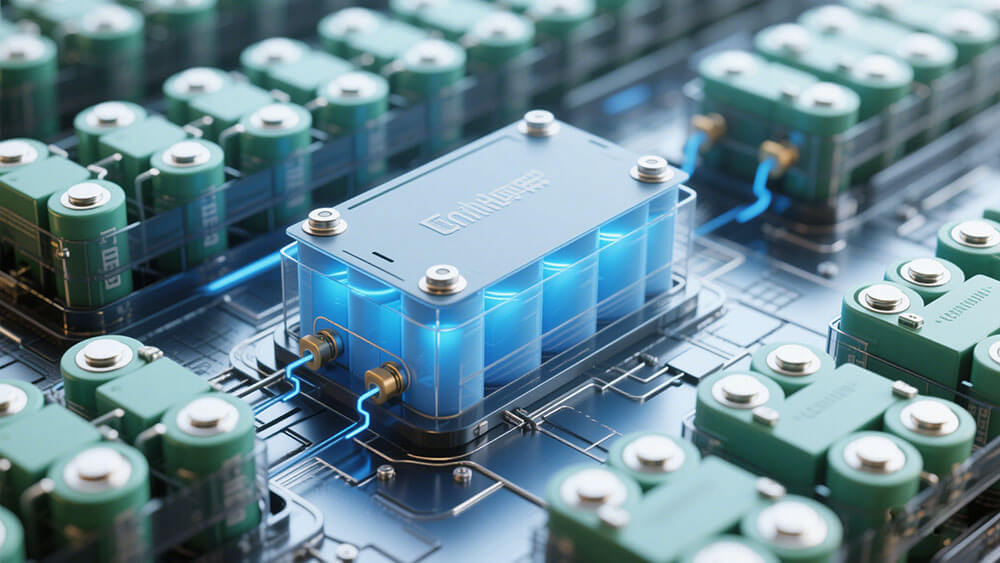
Part 4: Comparison of Data-Driven and Model-Based Methods
4.1 Accuracy and Reliability in SOH Estimation
When evaluating the accuracy and reliability of state of health estimation methods, both data-driven and model-based approaches offer unique strengths and limitations. Data-driven methods excel in leveraging real-world data to create predictive models. These methods analyze battery degradation patterns directly, making them highly effective for applications where real-time monitoring is essential. For example, artificial intelligence algorithms, such as neural networks, can model complex relationships in battery data, enabling precise predictions of capacity and performance.
In contrast, model-based methods rely on laboratory-calibrated simulations of lithium-ion batteries. These methods incorporate detailed physical and electrochemical models, providing insights into degradation mechanisms. However, their reliance on controlled conditions may limit their accuracy in real-world scenarios. For instance, variations in temperature or charge-discharge cycles can introduce discrepancies between model predictions and actual battery behavior.
Aspect | Data-Driven Methods | Model-Based Methods |
---|---|---|
Accuracy | High accuracy in real-world scenarios due to direct analysis of battery performance data. | High accuracy under controlled laboratory conditions but may struggle with real-world variability. |
Reliability | Reliable for diverse datasets, including noisy or incomplete data. | Reliable when operating conditions align with model assumptions. |
Adaptability | Adapts well to different battery chemistries and usage patterns. | Requires recalibration for new chemistries or operating conditions. |
Tip: If your application involves unpredictable operating conditions, such as robotics or infrastructure, data-driven methods may offer better reliability. For more tailored solutions, explore Large Power’s custom battery solutions.
4.2 Scalability and Computational Requirements
Scalability and computational efficiency are critical factors when selecting a state of health estimation method, especially for large-scale industrial applications. Data-driven methods demonstrate significant advantages in this area. By monitoring parameters like voltage and current, these methods reduce computational complexity. Empirical analyses reveal that data-driven approaches achieve up to 2,000 times lower latency and 27 times lower memory usage compared to simulation-based models. Additionally, their energy consumption remains negligible, making them ideal for battery management algorithms in energy-constrained systems.
Model-based methods, on the other hand, require extensive computational resources. Simulating the internal dynamics of lithium-ion batteries involves solving complex equations, which increases latency and energy overhead. This makes model-based approaches less scalable for applications requiring real-time SOH estimation, such as consumer electronics or security systems.
Aspect | Data-Driven Methods | Model-Based Methods |
---|---|---|
Computational Efficiency | Low latency and memory usage; negligible energy consumption. | High latency and memory usage; significant energy overhead. |
Scalability | Highly scalable for large-scale systems and real-time applications. | Limited scalability due to high computational demands. |
Implementation Complexity | Simple implementation with minimal hardware requirements. | Complex implementation requiring advanced computational infrastructure. |
Note: For applications like battery management systems in industrial environments, data-driven methods offer a clear advantage in scalability and efficiency. To learn more about optimizing your battery systems, consult Large Power’s custom battery solutions.
Part 5: Practical Considerations and Use Cases
5.1 When to Choose Data-Driven Methods
Data-driven methods excel in scenarios where you have access to extensive historical and real-time data. These methods are particularly effective for applications requiring real-time monitoring and adaptability to diverse operating conditions. For example, in electric vehicles (EVs) and hybrid electric vehicles (HEVs), data-driven approaches analyze charge-discharge cycles and temperature variations to predict the state of health with high accuracy.
You should consider data-driven methods when:
Data availability is abundant: These methods thrive on large datasets, including voltage, current, and temperature readings.
Real-time operation is critical: Data-driven models can process information quickly, making them ideal for dynamic environments like EVs and robotics.
Scalability is a priority: These methods adapt well to different battery chemistries and usage patterns, ensuring seamless integration across various systems.
Key Considerations | Why Data-Driven Methods Work |
---|---|
Real-time Monitoring | Machine learning models process data in real-time, ensuring accurate SOH predictions. |
Adaptability | These methods handle noisy or incomplete data effectively, ensuring robust performance. |
Scalability | Suitable for large-scale applications, including energy storage systems and industrial setups. |
Tip: If your application involves unpredictable conditions, such as robotics or infrastructure, data-driven methods offer the flexibility and reliability you need. For tailored solutions, explore Large Power’s custom battery solutions.
5.2 When to Choose Model-Based Methods
Model-based methods are ideal when you require a deep understanding of the internal dynamics of lithium-ion batteries. These methods rely on mathematical and physical models, making them suitable for applications where precision and insight into degradation mechanisms are essential. For instance, in medical devices, where safety and reliability are paramount, model-based methods provide the accuracy needed to ensure optimal performance.
You should choose model-based methods when:
High accuracy is non-negotiable: These methods simulate electrochemical processes, offering precise SOH predictions.
Controlled environments are available: Model-based approaches perform best under stable operating conditions.
Insight into degradation is required: By modeling physical processes, you can optimize battery design and usage.
Key Considerations | Why Model-Based Methods Work |
---|---|
Precision | Simulates internal battery dynamics for accurate SOH estimation. |
Degradation Insights | Provides a detailed understanding of battery wear and tear. |
Stability | Performs reliably in controlled environments, such as laboratories or medical applications. |
Note: For applications like medical devices, where safety and precision are critical, model-based methods are a reliable choice. Learn more about optimizing your battery systems with Large Power’s custom battery solutions.
5.3 Hybrid Approaches for SOH Estimation
Hybrid approaches combine the strengths of data-driven and model-based methods, offering a balanced solution for state of health estimation. These methods leverage the adaptability of machine learning with the precision of physical modeling, making them ideal for complex applications like EVs and energy storage systems.
Recent advancements highlight the potential of hybrid methods:
Qu et al. integrated particle swarm optimization and an Attention mechanism with LSTM to predict SOH and Remaining Useful Life (RUL) of lithium-ion batteries.
Venugopal et al. developed a method using independent recurrent neural networks, adapting to dynamic load conditions in EVs for accurate SOH predictions.
Li et al. designed a variable length time memory neural network, enhancing prediction accuracy through innovative gate connections.
Hybrid methods are particularly useful when:
Data and physical models are both available: These approaches utilize the best of both worlds for superior performance.
Dynamic conditions exist: Hybrid methods adapt to varying operating environments, ensuring reliable SOH estimation.
High accuracy and scalability are required: By combining techniques, hybrid methods achieve optimal results across diverse applications.
Call to Action: Hybrid approaches represent the future of SOH estimation. For customized solutions tailored to your needs, consult Large Power’s custom battery solutions.
Choosing the right method for estimating the state of health of lithium-ion batteries depends on your specific requirements. Data-driven methods excel in real-world adaptability and scalability, while model-based approaches provide unmatched precision and insights into battery degradation. Hybrid methods combine these strengths, offering a balanced solution for complex applications.
Method Type | Strengths | Weaknesses |
---|---|---|
Data-driven | High accuracy, practical, avoids complex chemistry | Dependence on historical data, prediction uncertainty |
Model-based | Theoretical insights, detailed analysis | High complexity, less practical for engineering |
Hybrid | Combines adaptability and precision | Requires both data and physical models |
Selecting the right approach ensures optimal performance, cost-efficiency, and reliability. For tailored solutions, explore Large Power’s custom battery solutions.
FAQ
1. What is the difference between NMC and LiFePO4 Lithium batteries in terms of SOH estimation?
NMC batteries offer higher energy density (160–270 Wh/kg), while LiFePO4 batteries excel in cycle life (2000–5000 cycles). Choose based on application needs.
Battery Type | Energy Density | Cycle Life | Platform Voltage |
---|---|---|---|
NMC | 160–270 Wh/kg | 1000–2000 cycles | 3.6–3.7V |
LiFePO4 | 100–180 Wh/kg | 2000–5000 cycles | 3.2V |
Tip: For industrial applications, LiFePO4 batteries provide better longevity. Learn more about Large Power’s custom battery solutions.
2. How do hybrid SOH estimation methods benefit robotics applications?
Hybrid methods combine adaptability and precision, ensuring accurate SOH predictions under dynamic load conditions. This enhances reliability in robotics systems.
Call to Action: Explore robotics battery solutions for tailored SOH estimation methods.
3. Why is SOH estimation critical for medical devices?
SOH estimation ensures battery reliability and safety, which are essential for medical devices. Accurate predictions prevent failures during critical operations.
Note: Discover medical battery solutions for optimized performance and safety.